The Business Value of Synthetic Data: Accelerating Growth While Reducing Costs
August 21,2023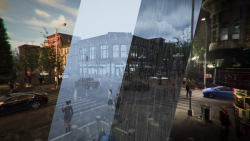
In the contemporary data-driven business landscape, acquiring quality data for machine learning (ML) and artificial intelligence (AI) applications is a top priority. However, real-world data can be challenging to collect, clean, and structure. That's where synthetic data steps in, providing numerous benefits and reshaping the way businesses interact with data. The four key value propositions of synthetic data are speed, control, business agility, and cost. Keep reading to understand each one in detail:
Speed: Rapid Iteration and Model Building
Time is of the essence in the business world, and synthetic data offers a significant speed advantage. Instead of spending weeks or even months gathering, cleaning, and structuring real-world data, businesses can generate synthetic datasets in hours or even minutes. This agility empowers them to build and iterate on their models quickly, enabling them to respond promptly to market changes, customer needs, or competitive pressures.
Furthermore, synthetic data allows businesses to run simulations and tests concurrently, accelerating the learning curve for machine learning models. This expeditious approach to model development can significantly enhance the business's ability to innovate and stay ahead of its competition.
Control: Construct Your Ideal Scenario
With synthetic data, businesses no longer need to wait for specific scenarios to occur naturally or spend exorbitantly to recreate certain conditions. Instead, they can generate any situation they want to test with synthetic data, creating thousands of permutations in seconds. This level of control allows businesses to run complex simulations and tests that might not be feasible with real-world data.
Imagine needing to test a self-driving car's response to a rare, hazardous condition. With synthetic data, you could generate thousands of iterations of that scenario instantly, allowing you to test and refine the car's responses without risking real-world accidents.
Business Agility: Embrace Change Effortlessly
Business needs change all the time. A new product may require changes in labeling data, altering hardware, or shifting ontologies. Adjusting real-world data to fit these new requirements often involves re-curating or relabeling an entire dataset—a time-consuming and expensive process.
On the other hand, synthetic data can be generated to fit the new requirements, incorporating changes in seconds. Whether you need different labels, different hardware configurations, or different ontology structures, synthetic data is flexible enough to keep up with your business's ever-changing needs.
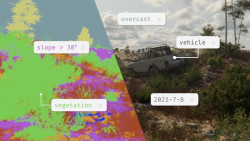
Cost: Increased Productivity and Lower Expenses
Synthetic data's speed, control, and agility all aggregate into lower costs. Faster model development times and fewer hours spent cleaning and structuring data means lower labor costs. The ability to generate ideal scenarios eliminates the need to spend on recreating specific conditions.
Moreover, solutions such as Bifrost can augment the productivity of the team by facilitating the development of projects in significantly shorter time frames. They enable engineers to handle more tasks, further enhancing cost efficiency and business output.
Conclusion
In conclusion, synthetic data offers incredible business value. By accelerating model development, granting control over scenarios, providing business agility, and reducing costs, it can empower businesses to thrive in the fast-paced, data-driven world of today. By integrating synthetic data into your data strategy, your business can leap ahead, delivering innovative solutions and maintaining a competitive edge.
Ready to generate data? Create a Bifrost account and start generating synthetic data now! Contact us at sales@bifrost.ai to enable access to the generative channels you need.